As data integrators and data curators of biomedical data, The Hyve’s specialists need to overcome a variety of data-centric challenges by using the FAIR principles as a leading approach. This blog aims to explain how data evaluation frameworks can benefit from a combination of FAIR metrics and quality key performance indicators (KPIs), and why you should consider making this a routine practice if you want to have a holistic view on data across the whole value chain and data lifecycle.
Making data FAIR can help improve data quality
“How good is your data?” This question has many possible answers. The outcome can change over time and depend on who is asking. Quality requirements, for instance the accuracy and completeness of your data, are usually directly imposed by the analytics tool of choice.
The conversation starter is usually the data readiness objectives set by your machine learning experts. FAIR principles do not, in themselves, cover the crucial aspects of intrinsic data quality or ethics and FAIR data are not per se high quality data. You could say FAIRness and quality are different aspects of data but the two are not totally unrelated. If you look at FAIR, it usually means you want to increase machine discoverability and (re)use of your data. For this reason, we recommend building a combination of FAIR indicators and quality KPIs, and to make this joint evaluation a routine checkpoint of any data management plan.
Note that the FAIR principles referenced here should not be confused with the concept of algorithm fairness, a relatively recent topic in machine learning, revolving around historical biases that may not be measured in most popular data quality frameworks.
If you are new to the FAIR principles, I suggest you start by reading this Nature article here.
FAIRness and quality are two different but not necessarily alternative aims. By defining the right intermediary objectives and understanding relevant elements of your data landscape and ecosystem of tools, both can be reached. FAIR data are Findable, Accessible, Interoperable and Reusable data. However, how FAIR a particular dataset can really be strongly depends on the context in which the data exist. The bar can always be raised and − in principle − the FAIRness can always be improved.
At The Hyve, we believe there are benefits to integrating FAIR recipes and objectives into a data quality framework. For instance, when trying to make health data machine-readable, the aim is to increase or facilitate external collaborations. Hence, we immediately think of a broader context for (re)use of the data. When the perspective is instead towards internal assets, the approach to ensure high quality data is usually reversed. One usually tries to have stringent gateways into data lakes and into those repositories where quality needs to be high to avoid “pollution”. You see how, when focussing exclusively on data quality, the purpose isn’t immediately to improve shareability of the data.
At The Hyve we believe that elements of data quality are implicit in the FAIR principles, especially R1. With regard to the relationship between FAIR and data quality, our founder Kees van Bochove said in a previous blog:
“It seems a mistake that the FAIR principles would not explicitly include these quality aspects, and people have called this out in the past, even asking for a ‘new letter’, for example the ‘U’ of Utility (now covered in the explanation of FAIR Principle R1). However, because the utility of data is so dependent on context, especially the intended use of the dataset, these topics may require guidelines and frameworks of their own.” Keep reading here.
When running a data quality assessment, the aim is to make sure your data is fit for a specific purpose. You may need to measure things like auditability, uniqueness, timeliness, et cetera. Some also include measures of the impact of data on strategic business goals.
What can FAIR do to help with data quality?
All things considered, data quality ultimately is a measure of the data being fit for a specific purpose: research, business, etc. The FAIR principles on the other hand can help unlock the potential for endless reuse. Paraphrasing the late Amrapali Zaveri, PhD, Postdoctoral Researcher at Maastricht University: “Increasing the FAIRness of your digital assets will increase their quality and improve potential and ease of reuse.”
Our advice is to understand the overlaps between the two available frameworks and then to develop an approach and measure what best suits your organization’s needs:
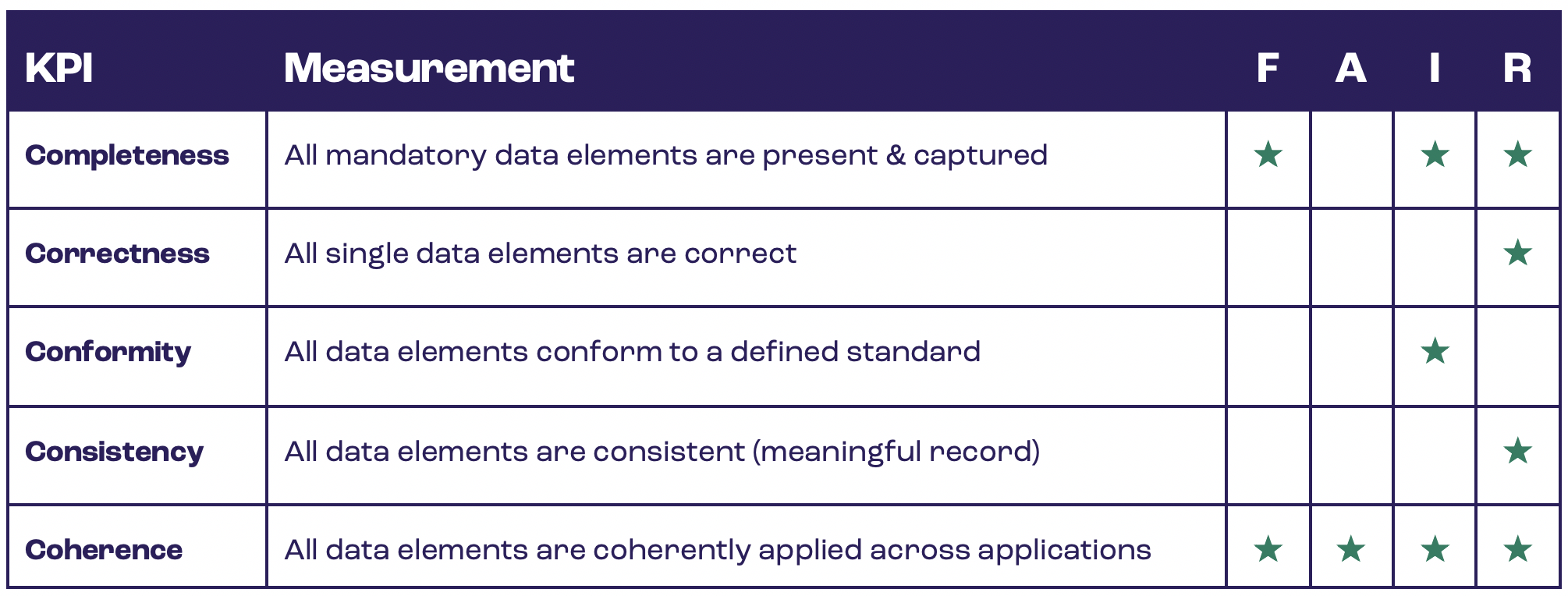
Five practical steps to add FAIR to Data Quality Frameworks
1. Discuss and define what FAIR means for your organization, and set goals for your short and long term needs.
2. Gather a factual representation of your data landscape, or at the very least a representative subset of data.
3. Measure Quality
Optimize FAIR measures by adding a custom, weighted- score, e.g. if your team has done a lot of work to curate and normalize data, but the results are still poor because some limitations are brought in by the tools for data analysis, you want to have this information reflected in the scores.
5. Compare results with goals
Both FAIR data and high quality can be achieved by understanding your data lifecycle. If you look at the overall data landscape with a long term utilization view, you open up the possibility of uncovering new value in your data for unexplored use cases.
FAIRness of data assets as the place to start and the end goal
FAIR helps achieve an enterprise-wide alignment of vision and guidelines to leverage company data as an asset. Such alignment, captured in a data strategy, ensures that different groups within the enterprise view data-related capabilities with consistency, reducing redundancy and confusion. Agreement on key metrics and success criteria across the enterprise, defining “success” and “quality” across all levels of interacting organizations, enables repeatability and consistency, reduces operational cost and optimizes performances, by creating a shared vision.
“Data is the factual currency for evidence-based policy making. Data travel a long journey, gaining value as they go, before they achieve their highest purpose.”- OpenDataWatch.