The importance and potential of the FAIR principles for the life sciences have quickly become apparent since their introduction in 2016. Many biomedical research organizations now not only strive to improve their data assets by following and trying to adhere to the FAIR principles but have also joined forces to support and further advance the original initiative. The implementation of the FAIR principles in practice requires evaluation of the FAIR level of data. This need for evaluation led to the generation of multiple Maturity Indicators and assessment frameworks. FAIR Metrics has been developed by FAIRsharing.org and the FAIR Data Maturity Model by Research Data Alliance (RDA). The Hyve has actively contributed to the Dataset Maturity Model (DSM) as part of the FAIRplus consortium. The conformance of a research dataset to these frameworks can be evaluated via a so-called ‘FAIR assessment,’ which can be executed either manually or by automated tools.
The Hyve has previously performed a comprehensive comparison of open-source FAIR assessment tools as part of a project with a top ten global pharma company. Since then, more automated tools for FAIR assessment have been released. To stay on top of the current landscape, we again performed a comparison of the available tools to reveal the strengths and limitations of each solution. In this article, we present and compare one manual and three automated open-source tools for FAIR assessment of a data resource, namely the Australian Research Data Commons (ARDC) FAIR Data Self Assessment Tool, FAIR-Checker, F-UJI, and FAIR Evaluation Services.
Evaluation of FAIR assessment tools using a CINECA synthetic dataset
The Hyve has been a member of the CINECA consortium since 2019. Part of the work around CINECA Work Package 3 (WP3) included the generation of synthetic datasets. A synthetic dataset is artificially generated and reproduces the characteristics and structure of an original dataset but does not contain any identifiable person/patient data. This means that it can be used in place of the original dataset for testing models without privacy concerns.
We performed the FAIR assessment on one of the CINECA synthetic datasets, namely the CINECA synthetic cohort Europe CH SIB (publicly available on Zenodo). The aim of the effort was two-fold: (i) to explore the functionalities of various FAIR assessment tools and compare their results, and (ii) to perform a brief FAIR assessment of the CINECA synthetic dataset.
There is an increasing number of frameworks and tools available to consider for a FAIR assessment, either manual or automated, with many of them being open-source. Manual tools such as the Data Archiving and Networked Services (DANS) SATIFYD and the ARDC FAIR Data Self Assessment Tool are mainly available in the form of online questionnaires. These questionnaires can be useful for triggering discussions at the initial stages of considering FAIR implementation or for identifying areas for improvement. Automated tools, such as F-UJI and FAIR Evaluation Services, are emerging, with many of them being under active development. The automated tools perform a number of tests starting from a dereferenceable URL to the data resource to be evaluated. They usually require minimum human effort to be implemented and are the most promising solutions for scalability, since they can automate the performed task within a reasonable timeframe and without requiring much human effort.
Multiple aspects can be considered when performing a comparative evaluation of these FAIR assessment tools, ranging from the selected framework and the number of tests performed on a dataset to usability characteristics, such as how easy it is to install or interact with the tool. Our comparative evaluation of the four open-source tools for FAIR assessment covered the following features: (i) visual representation of results; (ii) availability of documentation; (iii) downloadable format of the assessment results; (iv) the framework used for testing; (v) distinction between data and metadata in the performed tests; and (vi) scalability of the tool. Details of the evaluation are presented in Figure 1.
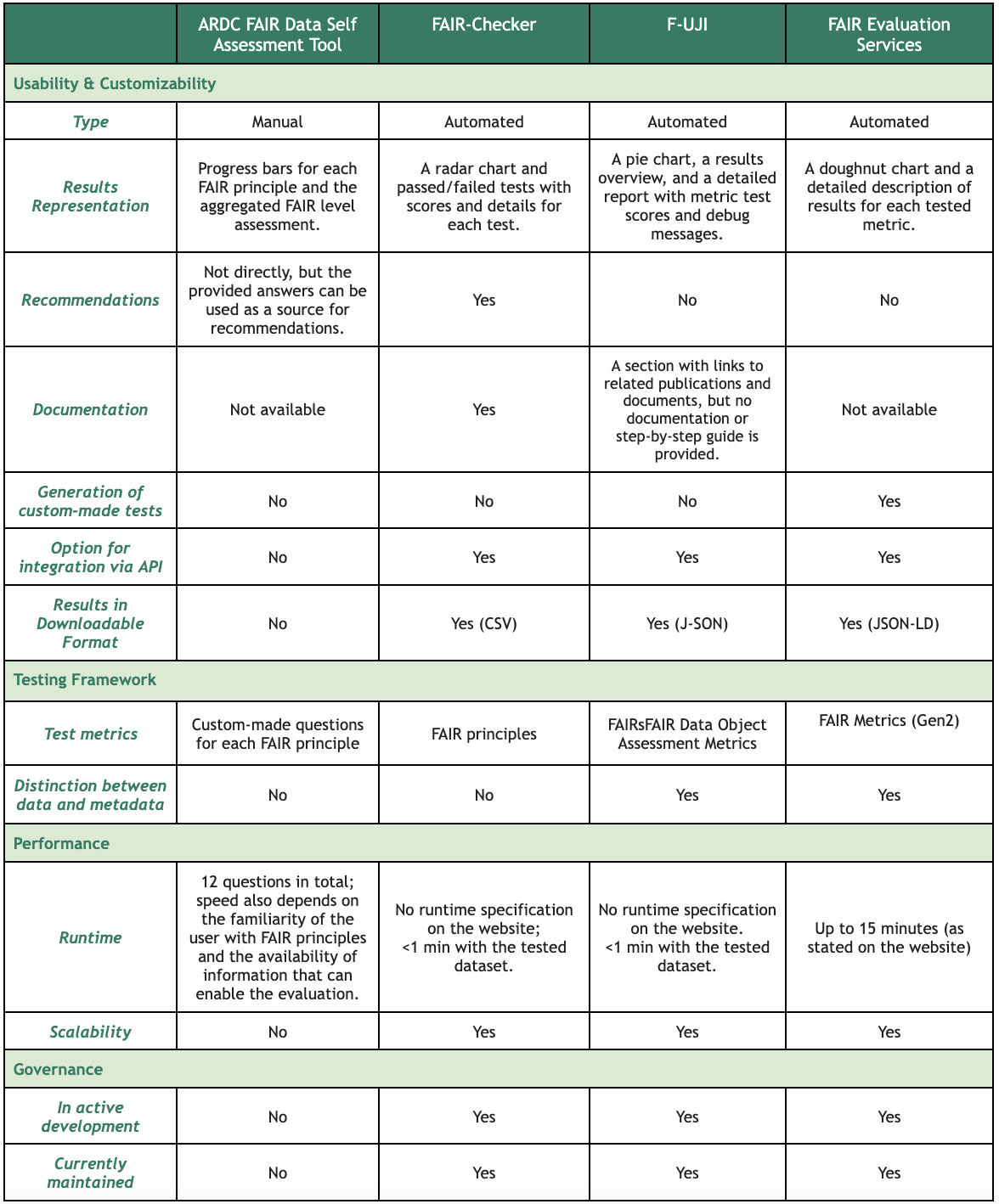
* Disclaimer: Some of the tools are under active development, thus the results of the above-described analysis might change over time.
FAIR Assessment Tools
- ARDC FAIR Data Self Assessment Tool
The FAIR Data Self Assessment Tool is an online questionnaire created by ARDC. It is, in principle, a guided approach to performing a manual FAIR assessment, and there is no need to provide a URL or upload the data resource for evaluation.
The tool contains a small number of questions (2-4) regarding some of the sub-components of each FAIR principle, adding up to 12 questions in total. A drop-down list with a pre-determined set of answers is provided for each question. Once an answer is selected, green progress bars are updated in real-time, indicating the score related to each FAIR principle. There is also a ‘total score’ progress bar that represents the overall assessment score across all principles.
For each question, there is a detailed description available. However, the tool’s website does not provide any other form of documentation. Being familiar with the FAIR principles and the resource being tested allows for a quicker and more comprehensive evaluation.
The ARDC FAIR Data Self Assessment Tool does not explicitly specify which FAIR sub-principles are covered by each of the available questions, and there is no clear breakdown in the questions to enable a separate assessment of data and metadata of the tested resource. The tool is also not scalable as it requires human input to answer the predefined questions and be able to get an output.
- FAIR-Checker
FAIR-Checker is an automated tool for FAIR assessment of online resources, such as datasets or software applications. It has been developed by the Interoperability Working Group of the French Institute for Bioinformatics. This assessment tool is publicly available online and requires no registration or authorization to be used.
FAIR-Checker contains a section for checking datasets. The user just needs to provide a valid PID or URL of the dataset’s landing page (i.e. URL/DOI) to be checked.
The output of the FAIR assessment is visually presented with a radar chart that illustrates normalized evaluation results (on a scale from 0 to 100) for the four FAIR principles. A table with detailed scores, results, log messages, and recommendations for each performed test is also provided. However, it is not clearly indicated to what extent the scores in the table correspond to those in the radar chart.
The runtime for an assessment of a dataset is not specified in the documentation. In our case, testing for all metrics for the CINECA synthetic dataset required less than a minute.
FAIR-Checker tests do not make a distinction between tests performed on data and metadata. Also, not all FAIR principles are considered, such as some of the Findability (F3, F4) and Accessibility (A1.2, A2) sub-principles.
- F-UJI
F-UJI is a publicly available automated tool that has been developed by FAIRsFAIR and uses the FAIRsFAIR Data Object Assessment Metrics. The only required input is a valid PID/URL of the dataset’s landing page. By default, F-UJI uses DataCite to retrieve JSON metadata based on the provided DOI. This option can be unchecked in the Settings options. Then the landing page URL will be used instead. It is also possible to provide a metadata service point as an input and specify its type (i.e. OAI-PMH, OGC CSW, SPARQL).
The runtime is not specified in the documentation, but there are progress messages provided while the assessment is running, informing the user which maturity indicator is being tested. In our case, testing all metrics for the CINECA synthetic dataset required less than a minute.
Once the assessment is completed, a visual representation of the results is provided as a multi-level pie chart. Unfortunately, the pie chart in the tested version is not interactive; it is not clickable and does not provide extra information via labels.
A detailed report specifying the tests that were performed and their corresponding ‘FAIR level’ is provided. The ‘FAIR level’ (i.e. initial, moderate, and advanced) is represented by colored checkmarks. Also, debug messages are available for each test, enabling the end user to independently check and evaluate the test outputs.
- FAIR Evaluation Services
FAIR Evaluation Services has been developed by the FAIR Metrics group (fairmetrics.org) and FAIRsharing and considers the Gen2 FAIR Metrics. There is an option to test only one specific principle (e.g. Findability), all four FAIR principles, or to use custom-made maturity indicators tests (defined using SmartAPI). Tests for some of the Reusability principles (i.e. R1.2, R1.3) are not included in the Gen2 FAIR Metrics and, thus, are not tested with this tool. The user needs to provide a Globally Unique Identifier of the (meta)data (e.g. DOI), along with an ORCID and a title for the evaluation.
After filling out these details and hitting the ‘Run Evaluation’ button, it can take up to 15 minutes before the tool provides a detailed output of the performed tests.
The output contains a summary of the assessment (i.e. resource tested, number of successful and failed tests, and a link to the JSON response of the assessment that can be stored). An interactive doughnut chart specifies the number of tests that passed or failed, followed by a detailed list of the passed and failed tests, which are indicated with green and red color, respectively.
For a failed test, log messages from this test are also displayed. However, there is no ‘human-friendly’ version of the output. This may prove burdensome for a user that is less familiar with the underlying maturity indicator tests and the code used to run them. In addition, the log messages don't always provide a clear answer as to why the resource failed the test.
Comparison of tool features
We compared and rated the selected tools using the following key domains (Figure 2): (i) Usability & Customizability; (ii) Testing Framework; (iii) Performance; and (iv) Governance.
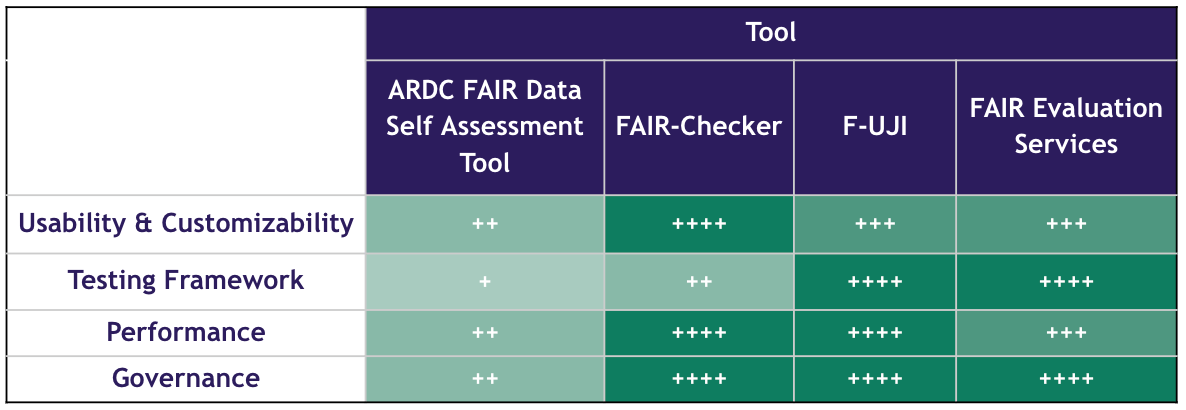
The four tools use very different frameworks for FAIR evaluation and present their outputs by different means. The level of familiarity with FAIR principles as well as computer literacy of the user needs to be taken into account, as it can be a real challenge to understand the output of assessment tools. Also, some of the tools consider data and metadata separately to evaluate the FAIR level of a resource, while others perform an aggregated evaluation without distinguishing between the two. This might lead to inconsistent results, depending on the tools used.
Visual representations of the results are provided by all tools, but not all are interactive or intuitive. Most of them could definitely be improved upon to provide a clearer and more user-friendly output of the evaluation. The assessment results provided by the automated tools are also available in downloadable format and can be stored if needed. With FAIR-Checker, it is possible to export the results table as a CSV file. FAIR Evaluation Services and F-UJI enable output storage in JSON-LD and JSON format, respectively. For the ARDC FAIR Data Self Assessment Tool, there is no option to export the results of the assessment.
Considering the ability of the tools to provide recommendations on how to improve the FAIR level of a data resource, only FAIR-Checker contains a dedicated field in the results section with suggestions based on failed tests. In the ARDC FAIR Data Self Assessment Tool, the predefined set of answers for each question provided can be used as suggestions to improve the FAIR level of a dataset. For example, when assessing provenance information, this tool gives the following options: provenance information can be completely missing, partially recorded, fully recorded in a text format, or fully recorded in a machine-readable format. Based on the existing status of the data resource and the need for improving the aspect of data reusability, efforts towards providing provenance information could be driven using these options.
In terms of documentation, FAIR-Checker provides a well-structured resource with a landing page, sections, and helpful visuals. For F-UJI, there is a section with links to publications and documents. There is no documentation or step-by-step guide for the web application, but it is straightforward to use and with a user-friendly interface. Other helpful resources which are not listed on the F-UJI website (e.g. demo video) can be found when searching online. On the other hand, no documentation is available for the FAIR Data Self Assessment Tool and FAIR Evaluation Services.
Unlike the manual FAIR Data Self Assessment Tool, the three automated tools are scalable. FAIR-Checker provides an API that allows users to check one or multiple metrics. For F-UJI, there is a REST API and an R client package. FAIR Evaluation Services also provides an API. However, evaluating a single resource using the web interface can currently take up to 15 minutes. This slow runtime could be an obstacle to scalability.
CINECA synthetic dataset FAIR evaluation
For Findability, the various assessments of the CINECA synthetic dataset provided inconsistent results. Take, for example, the tests related to the persistence of the identifier. F-UJI recognizes and uses the DOI as the data identifier, while FAIR-Checker and FAIR Evaluation Services rather use the download URL as the data identifier (and DOI as the metadata identifier). Hence, test results differ between the tools.
F-UJI managed to retrieve relevant metadata for the CINECA synthetic dataset (available as JSON-LD), while the FAIR-Checker and FAIR Evaluation Services used the DOI of the tested dataset to perform this test.
The CINECA synthetic dataset scored high in all tools on Accessibility. Not surprisingly: the dataset has a defined data and metadata protocol.
The Interoperability of the CINECA synthetic dataset could be enhanced in multiple ways, including the selection of a linked data format (e.g. RDF) for data representation and the use of terms from existing vocabularies and ontologies to define the data elements.
The last component of the FAIR principles, Reusability of the CINECA synthetic dataset, is fulfilled by having a standard machine-readable license attached to the data (i.e. Creative Commons Attribution 4.0 International) and using metadata standards, such as the DataCite Metadata Schema and Dublin Core. However, formal provenance metadata that considers entities from the PROV–O ontology, for example, is unavailable.
Overall, the dataset has an advanced ‘FAIR level’ in terms of its metadata but the ‘FAIR level’ of data could be improved in many ways. For example, by using a knowledge representation language (i.e. any form of ontologically-grounded linked data).
How to choose a tool
Selecting the right tool for a FAIR assessment is not straightforward and will depend on multiple factors, including, but not limited to, the scope of the assessment, desired functionalities and output, level of understanding of the FAIR principles by the end users. It is important to keep in mind that the results of an assessment performed on the same data resource can differ between the available tools, since they may define different sets of indicators or tests for assessment. In addition, although some FAIR principles are applicable to both data and metadata, some tools and frameworks do not provide separate metrics for the two components. By providing and using different tests or indicators when we perform a FAIR assessment of a dataset, it becomes easier to distinguish what is assessed and have a more comprehensive output. Another challenge for the different tools is clearly the ability to detect the resources to be assessed via automated tests. For example, license information might not be retrieved by the tool, although it is mentioned in the resource. Overall, a deeper understanding of the functionality of each tool is necessary before we can select the most suitable one for a particular use case.
Here are some points for consideration on how to select the right tool for FAIR assessment of your data:
Do you want to trigger a discussion within your team? A manual assessment using a questionnaire might be relevant in this case.
Is your dataset deposited to a public repository? This might be a requirement for some automated tools.
Do you consider evaluating sensitive data? The details on whether a tool stores the evaluated data (or what types of data it stores) are of paramount importance to opt for or reject a tool. Privacy concerns can also result in the incapability to utilize tools with desired functionalities.
Do you want to assess a FAIRification process? In that case, you could perform a FAIR assessment before and after FAIRification and compare pre- and post-FAIRification assessment scores.
Are recommendations for improving the ‘FAIR level’ of a dataset needed?
Do you need to define customized maturity indicator tests? This is not possible with most tools.
Is the integration of the tool via API required?
The availability of multiple frameworks for FAIR assessment provides the flexibility to each time select the one that fits our purpose. At the same time, it leads to inconsistencies between evaluations. For the FAIR community, we think it is important to create partnerships that work towards harmonization of the existing frameworks rather than focussing on the generation of new ones.
In conclusion, FAIR assessment is not a straightforward activity. It requires a thorough understanding of the FAIR principles, evaluation frameworks, and available technologies. Also, if the overall aim is to work towards establishing a ‘FAIR organizational culture,’ assessing the FAIR level of data per se is not sufficient. Understanding and assessing the data policies, and level of knowledge and experience with the FAIR principles within an organization are all valid and important areas to explore. The Hyve can provide expertise in selecting the appropriate criteria and tools for a FAIR assessment based on the needs within an organization, support FAIR assessments and provide recommendations.
Acknowledgements
The Hyve acknowledges support from the European Union’s Horizon 2020 Research and Innovation Programme under grant agreement No 825775, and the Canadian Institute of Health Research under grant agreement No 404896, Common Infrastructure for National Cohorts in Europe, Canada, and Africa (CINECA) project.