In the January 2020 special issue on emerging FAIR practices of the Data Intelligence journal, metadata is defined as “any description of a resource that can serve the purpose of enabling findability and/or reusability and/or interpretation and/or assessment of that resource”. However, metadata itself is also a digital resource that should be FAIR. So this definition creates a Russian doll effect where you can keep zooming in and make your data and the context of your data more granular and semantically precise. Or you could compare it with a fractal, although in practice these zoom levels are not infinite, as we will see in a moment.
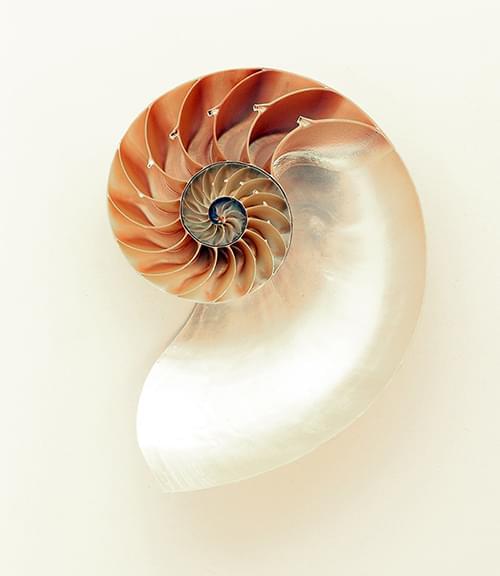
The purpose and scope of FAIRification
When implementing the FAIR principles in a large organization such as a big pharma company or an academic hospital, it is important to be aware of this scaling effect, so as to be able to articulate the right ‘zoom level’ for a given purpose. As the saying goes “one person’s metadata is another person’s data”.
For management purposes it can be enough to get some quantitative insights in the amounts of data (digital objects / data assets) available in a particular area of the company - for example the combination of a certain medical indication and a class of measurements (‘omics’), or just the number of patient years in trials with a certain drug or target. This can then drive key performance indicators (KPIs) and metrics to inform strategic decisions.
On the other hand, scientists looking for datasets to answer a specific question may have a long list of detailed context information elements they need to know about a digital resource, in order to assess whether a certain dataset is helpful to answer the research question at hand. If researchers can consistently find and access these elements and retrieve the correct datasets easily, rather than having to ask around or look for it in folders and team spaces, this can greatly enhance their productivity. This in turn can speed up the research & development process.
In between those extremes, there are many different possible paths through the (meta)data fractal that people and machines can take in order to generate the insights they need. Data governance is all about capturing these distinctions, and making them explicit in the company’s data assets. But to link this to the company’s business goals and form a data strategy, the target stakeholders and their purposes need to be clear. Clarifying the purpose can also prevent disappointments: for example, setting up a catalogue of data assets leveraging Dublin Core metadata can be a good start, but is likely not be enough for both examples mentioned above.
The effort required and the time horizon needed to demonstrate results will vary greatly. Take the examples I mentioned: an overview of data assets can be made in a few months (The Hyve offers this service under the name ‘data landscape’). At that point, you can already start tracking said KPIs. On the other hand, the increased speed and turnover in the drug discovery & development lifecycle, thanks to better stewardship of corporate data assets, will take several years to materialize. These are better measured by proxies of scientific productivity such as time spent on retrieving and massaging data versus generating and interpreting results. Or, as suggested by colleagues from Bayer and Roche, by activating these FAIR data assets on the corporate balance sheet.
FAIRification versus FAIR play
Next to purpose and scope, there is another dimension that is extremely important for understanding the risk/reward-ratio of FAIRification that has its own effect on the ‘zoom level’ in the (meta)data fractal and the effort required. This has to do with the difference between ‘FAIRification vs FAIR play’, as Philippe Marc from Novartis put it at a conference last year: FAIRifying the data a posteriori, versus producing FAIR data natively. Let’s look at a concrete case to explore this further.
A nice example of zooming in quite deeply on the (meta)data fractal is the exemplar from our Oxford colleagues Philippe Rocca-Serra and Susanna-Assunta Sansone. They took a dataset from the rose genome publication in Nature Genetics and fully encoded it in Resource Description Framework (RDF) leveraging a number of public ontologies. The figure below illustrates the various steps they took to transform one Excel file to unambiguous machine ‘understandable’ data - another way to put it would be that they made the data fully ‘AI-ready’. See also our growing FAIRplus cookbook for more details. However, the linked Jupyter notebooks that were used to clean up and annotate this Excel sheet make clear that this was a significant effort. And this was just 1 out of the 10 supplementary datasets attached to this paper.
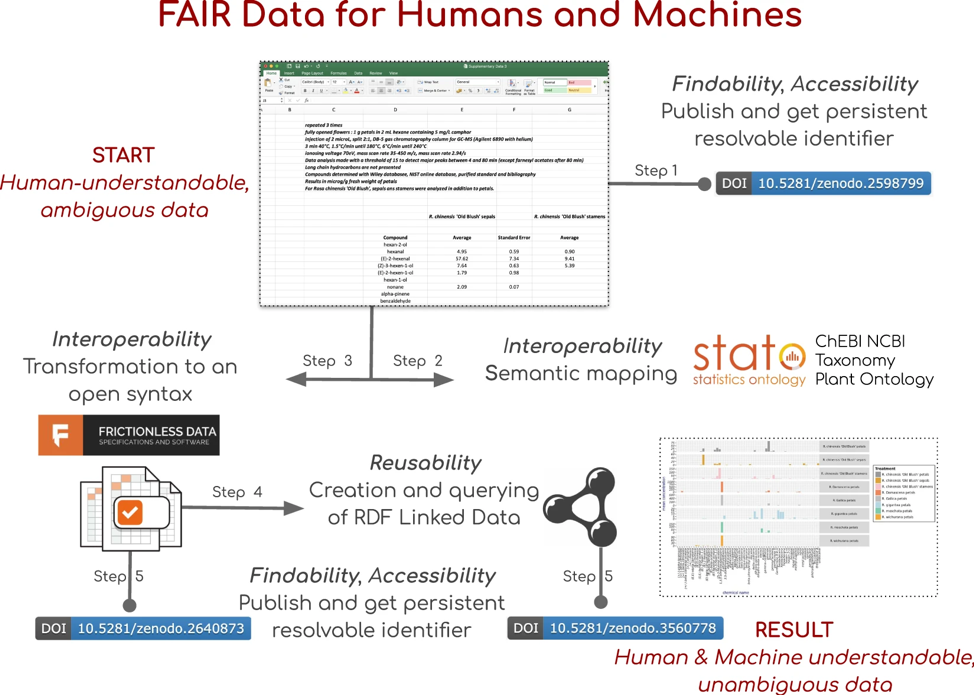
Why does retrospective FAIRification usually require a lot of effort? Consider that the Excel sheet provided here, like most scientific datasets, is the result of a long process (data lifecycle), with steps like Experiment design & planning → Experiment execution → Data processing → Data analysis → Data publishing. But we are only looking at the end result of the last step, and if you look at the example Excel file, you will find that elements of the other steps (e.g. experimental design) are written into it as textual comments. Useful for the human eye, but not exactly ‘Metadata for Machines’. If you want machines to be able to find and use these data, then the (meta)data need to be structured and semantically explicit.
So the reason why the overall effort is so large, is that one has to effectively ‘reverse engineer’ all these steps from the Excel sheet and the text in the paper, which at this point can only be done by humans - machine learning would stand no chance in successfully doing this for multiple reasons, one being that getting enough training data would simply be too costly.
The solution, in terms of scaling up FAIRification, is to go back to the earlier steps in the process, and change them to produce FAIR data too. Although this approach to FAIRification also takes a significant amount of work, it is at least scalable. Once a lab instrumentation setup has been set up to generate FAIR data at source, it can be used routinely (FAIR play) and result in systematic annotation of all datasets that are generated through this instrument. The same goes for computational pipelines, which can be ‘FAIRified’ to output their versions, parameters etc. as machine readable metadata. Study designs, including for instance clinical trial protocols, can be made FAIR directly from the start of the project and linked to the resulting datasets. As a final step, there are several standards for representing biomedical data for re-use and analysis.
How do you get started with the implementation of FAIR play? DTL and GO-FAIR have introduced the concepts of Bring Your Own Data (BYOD) and Metadata for Machines (M4M) workshops, in which we take a number of existing data assets or a specific class of datasets, and determine the right level of metadata for a given purpose. This can then be used as a starting point to define metadata conventions, choose which of the existing standards to use, and eventually implement these in all data generating processes. The Hyve offers both BYOD and M4M workshops as well as semantic modelling and data FAIRification services, to support your organization in implementing the FAIR principles.
Although it may be necessary to start with retrospective FAIRification on some datasets, in the long run, FAIR play is the only way forward.