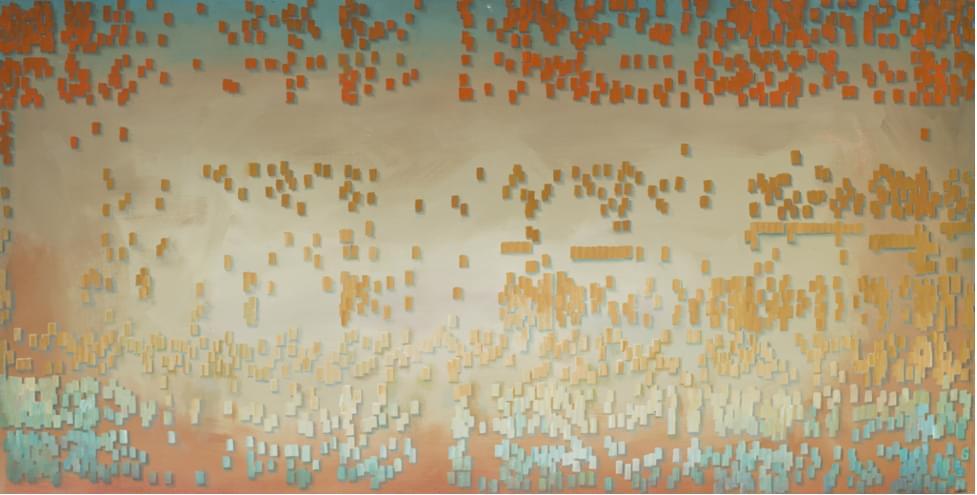
Interoperability between healthcare standards remains a key goal for open biomedical science in general and for EHDEN and The Hyve in particular, as described in a recent EHDEN blog post (and deliverable). We are working on many fronts here, such as the standardization of genomics data in GA4GH and CINECA, imaging data (see DICOM) for AI in PANCAIM, interoperability of healthcare data using HL7 FHIR, clinical trials data from pharma and non-profit organisations in CDISC, metadata and discoverability in RDF / JSON-LD using OBO Foundry ontologies and schema.org, and − of course − standardization of medical observational data using OHDSI OMOP. Even this seemingly impressive list is just scratching the surface, because as soon as you get into the weeds of biomedical data standardization, you realize that SNOMED-CT (used to indicate conditions in most of the above data models) isn’t just a terminology system but a full ontology, that some types of biomarkers such as flow cytometry measurements are difficult to model in LOINC, et cetera, et cetera.
In practical terms, perhaps the most promising development of all is the increasing alignment of HL7 FHIR and OHDSI OMOP. Both standards have gained a lot of momentum right now, especially in (research) hospitals, albeit from quite distinct driving forces. Let’s look at both of the standards briefly. By the end of the blog, I’ll give a perspective on the next steps that will be taken.
The rise of FHIR
HL7 FHIR has really taken root in the United States, thanks to the pioneering work of Argonaut and other accelerator projects such as Da Vinci, and implementation drivers such as Apple HealthKit with its large list of hospitals that synchronize medical data to the iPhone. More importantly, it is now directly cited in and aligned with the U.S. government mandated standards. I have written about this before, but it is exciting to see that the ONC core data set, the USCDI, is now directly aligned to the US Core FHIR profile. The same goes for coding systems such as those bundled by NLM VSAC. This means that American healthcare organizations that implement the US Core FHIR profiles in their key systems are well on their way to meeting government mandated standards. Although in Europe and elsewhere in the world, FHIR isn’t yet as popular or mainstream as in the U.S., the community is growing very fast. For example, in The Netherlands the MedMij FHIR standards have been developed for exchange of healthcare data and for synchronizing those data to personal healthcare apps. The FHIR community also regularly holds connectathons: events that address specific interoperability or connectivity issues related to FHIR. More on that later in this article.
The momentum of OMOP
For its part, the OMOP standard developed by the OHDSI community has seen a rapid rise in adoption, driven mostly by a relentless focus to scale up and improve the trustworthiness of global medical evidence generation through observational research. A number of high profile publications, such as an article in The Lancet on the effectiveness and safety of hypertension medicines and publications related to COVID-19 phenotyping, RAS blockers, and the safety of hydroxychloroquine, have all established the feasibility of the OHDSI approach. The paper on hydroxychloroquine also directly informed EMA statements and is cited as best practice in pharmacoepidemiology. The underlying open science driver for the OHDSI studies and approach is “a paradigm shift from single study and single estimate medical research to large-scale systematic evidence generation” (see the Book of OHDSI). This involves the systematic application of proper statistical designs, control hypotheses and methods such as propensity score stratification, as detailed in this paper. But, as enumerated in the LEGEND principles, it also involves open-source software and methods, pre-registration of study protocols, and of course the conversion of many databases globally to the OMOP Common Data Model so analyses can be run in multiple healthcare databases.
Meeting of the giants: FHIR and OMOP
It is on this last topic that FHIR and OMOP meet! The FHIR community has an increasing interest in facilitating secondary use of healthcare data for research (BR&R) and improving outcomes (value based healthcare, learning healthcare system, etc.) (Vulcan). On the other hand, the OHDSI community and projects like EHDEN and H2O face the challenge to scale up the conversion of routinely generated healthcare data, increasingly modeled in FHIR, directly to OMOP. So the logical next step is to facilitate the periodic conversion from routine healthcare data represented in FHIR into OMOP, by providing tooling and best practices for this mapping. Moreover, this mapping paves the way for other interoperability scenarios. For example, using FHIR as an intermediary between CDMs, as proposed by CAMP FHIR, or even going the reverse route of exposing OMOP as FHIR (see OMOPonFHIR) such as using OMOP CDM data to train or run machine learning clinical decision algorithms that have been written for compatible FHIR resources.
Mapping challenges: lessons from the FHIR connectathon
So what did we learn from the recent 27th FHIR connectathon and what is next? In the Vulcan Real World Data track in the connectathon, we mainly focused on a specific interoperability use case contributed by the FDA, namely the conversion of FHIR data to CDISC SDTM to facilitate submission of Real World Data (healthcare data represented in FHIR) to the FDA. Key learnings from this effort directly apply to mapping FHIR to the OMOP CDM, such as the wide variety of resources (MedicationStatement, MedicationRequest, MedicationDispense, MedicationAdministration, etc.) used ‘in the wild’ by EHR vendors to represent various medication events that in CDISC SDTM would go into the Concomitant Medication (CM) domain but in OMOP belong to the DRUG_EXPOSURE table. Or even the representation of the medication definition itself. For example, if you want to indicate that someone received the Pfizer/BioNTech COVID-19 vaccine, would you use the RxNav, UNII, EMA, PubChem, ChemIDplus, CAS, DrugBank, Wikidata code, just the English WHO INN tozinameran, a product name like BNT162b2 or Comirnaty, or even the mRNA sequence? And yes, this is still an incomplete list of identifiers for the product and doesn’t even describe the injection form and dosing yet, for which there is a whole other set of ontologies and terms. Then there are the other major OMOP domains, Conditions, Procedures and Measurements, which have similar complex terminology landscapes. And you can safely assume that just mapping basic demographics data to the Person and Observation Period domains isn’t going to be trivial either. Clearly, the biomedical community can benefit greatly from joint efforts between communities like HL7 and OHDSI.
What is next?
And this is exactly what we are going to do! As announced in the HL7/OHDSI collaboration, we will be working on aligning these communities and their respective standards. Much of it is still in flux but an obvious task will be to write a FHIR Implementation Guide that provides best practices for mapping FHIR resources to OMOP. The Hyve will be involved with that project/process of course, and as we have published before this is key to the interoperability goals of EHDEN and H2O projects as well. Keep your eyes open for further developments. And if you want to collaborate on this topic, please get in touch using the form below!